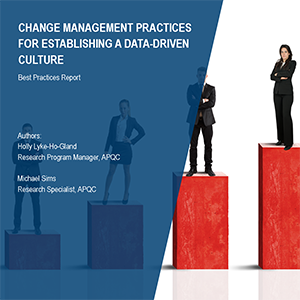
Tips for Constructing a Data-Driven Culture
By Holly Lyke-Ho-Gland
Analytics and data-driven decision making have become something of a holy grail for modern organizations. In December 2015, APQC conducted its annual survey to understand the common challenges and priorities of process and performance management practitioners in 2016. The survey covered a wide array of topics including data and analytics. Though most organizations have embarked on their analytics journey, many continue to struggle with embedding analytics into their business.
Two core needs emerged from the survey challenges:
- Establishing a data-driven culture
- Providing performance measures and ROI on analytics
There are many pre-requisites to an organization becoming data-driven, but none is more critical than having a culture that enables and encourages objective, data-informed decision making. To find answers to those challenges, APQC conducted research on change management best practices for establishing a data-driven culture.
Establishing a Data-Driven Culture
In previous research on change management, Transformational Change—Making It Last, APQC found that the key components of managing or rolling out any change, especially one that encompasses an entire organization, are:
- Ensuring the change has the necessary skillsets (technical expertise, domain expertise, and soft skills)
- Using an array of engagement tactics to generate buy-in and change behaviors
- Engaging employees in the process
In the case of data-driven decision making, the organization has to apply these practices when establishing analytics capabilities within the organization.
Ensure a Balanced Mix of Business Acumen with Technical Expertise
Regardless of whether the organization uses a centralized, function-specific, or federated model, any analytics program relies on three realms of expertise to be successful:
- Domain experts—They understand the business, can define the problem, understand what needs to be solved, and know how the insights are going to be used. Domain experts are responsible for using the insights derived from the analysis.
- Analytics experts—They are someone who knows the limitations and possibilities of analytics. Analytics experts are responsible for building the models.
- Data management experts—They are someone who knows where to get the data and what it means. Data management experts are often based in IT, data management experts are responsible for managing and cleaning the data.
Without domain experts, organizations might answer the wrong question exceedingly well. Without the analytics, organizations cannot get the insights they are looking for. Without the data people, organizations can define the problem perfectly, but have no way to extract the right data for the analytics. The collaboration between these areas of expertise not only ensures analytics projects are successful it also helps cross-pollinate concepts and terminology. Over time, the business and decision makers understand what analytics can and cannot be used for and the analytics teams understand and explain what they are doing in business terms.
Best-practice organizations also include interpersonal or soft skills in their analytics teams. Given the need to change behaviors and communicate with decision makers, analytics teams also need several soft skills—communication, facilitation, coaching, and storytelling.
Use an Array of Engagement Tactics
Transformational change extends to all parts of the organization and requires a major shift in behaviors. Furthermore, every business function or decision maker is not the same, and support is not one-size-fits-all. The use of multiple solutions is the only way to effectively support customers and stakeholders. Best-practice organizations use a broad mix of engagement techniques and service offerings for their analytics teams. Services provided include access to raw data, self-service dashboards, topical and segmentation analysis, one-on-one and group coaching to extract key insights related to specific questions to address business needs, as well as project design/implementation of the recommendation.
The best method for initial engagement tends to be helping the business understand its problem and how the recommendations from the analytics team and the models can help understand the trade-offs and impacts. For groups that are newer to data-driven decision making, analytics teams can provide access to self-service dashboards and work with the team to coach them on using the dashboard and help them understand what types of questions they want answered. The combination of self-service dashboards and regular coaching helps in determining what the team needs and the right way to get answers to their questions. This approach also makes employees more comfortable with data, ultimately reducing resistance.
Engage Employees with an Iterative Analytics Process
Best-practice research indicates that one way to overcome resistance and create a culture change is to engage employees in the process. This helps people feel that change is not simply something that is happening to them, but something they are creating. This ultimately gives them a sense of ownership and successfully achieves buy-in.
Using an iterative process for analytics projects helps engage employees in the process, particularly during the scoping and refinement phases. There are two primary considerations when kicking off an analytics project: one is what questions need to be answered and the other is what data you need to answer the questions. The analytics team needs to understand not just the question, but also its value or purpose to determine the fit of the available data or if capturing the information a different way could answer the question more effectively. Based on what the analytics team knows about data and its applications in modelling, it can provide recommendations on how the data can be used or better captured (i.e., what questions are being used to gather information) to optimize for business needs. To accomplish this aspect of the effort, regular interactions with decision makers through two-way collaborations are crucial.
Measuring Analytics
Measures not only help the organization monitor the success of its analytics programs, but helps embed a data-driven culture. Best-practice organizations keep the emphasis on change objectives through targeted measures of success to help reach long-term goals, one of them being culture shift. This enables the organizations to indicate value to the organization, keep stakeholders engaged, and make adjustments to the program as needed. The being said, understanding that you need measures and a cycle of structured review is easier said than done.
Use Measures that Capture the Value to the Business
It is crucial to use measures that decision makers find important. These typically include business performance measures such as cost, customer retention, and cycle time. By doing this, you measure the difference in a manner that demonstrates the value of analytics. Typically, best-practice organizations use a combination of measures in three primary categories: performance measures, business results, and adoption or growth measures.
Behavioral Change Measures
Since adopting analytics and shifting to a data-driven culture is about changing behaviors and norms, the majority of organizations in this study use behavioral change measures. These measures help monitor the adoption rates or changes in norms and practices within the organization. They also monitor the organization’s use of analytics outputs to support its decision making. Hence, the relevant measures include:
- Action items—These include the number and types of actions taken based on the analytics. This information helps outline the value of analytics and the ability of decision makers to act upon them.
- Utilization or consumption—This measure includes tracking the consumption or downloads of analytics outputs, either through self-service dashboards or repositories.
- Number of service requests—This includes both repeat and new requests for projects or analytics. Repeat business indicates the value of the insights provided for decision making, while new requests indicate the growing awareness of and value to the organization.
- Number of employees requesting training—This measure tracks the requests and outcomes of formal and informal analytics training. This information indicates the increase and adoption of analytics skills throughout the organization.
Analytics Performance Measures
Performance measures are focused on how well the analytics program is accomplishing it goals, which include:
- Prediction or model accuracy—This is how close the prediction of the changes in activity X would be if you took Y action(s).
- A/B comparatives—This means comparing the differences where the organization uses experimental design and compares where the organization uses insights from predictive models for a segment or smaller project to test its results (A) and uses its old models for the rest of the processes or organization (B).
- Cost/benefit analysis—The analysis measures the revenue or cost savings of the projects compared to the resource investment.
- Stakeholder satisfaction—This is the most qualitative measure, which helps understand how valuable the contributions are to decision makers. Consequently, stakeholder satisfaction will also typically identify areas for improvement or strengths to focus on.
Business Performance Measures
As noted earlier, it is vital to use measures that decision makers find important—typically business performance measures such as revenue, costs, customer retention, or cycle time. Often times, these business performance results are linked to specific analytics projects. This will give insight to overall business performance gains.
As with any cultural shift, moving towards a data-driven culture is a people-centric activity that requires sound change management tactics ranging from structured collaboration to targeted measures that track success. Effective change is not a one-off action; rather, organizations need to build engagement, communication, and even measures into the overall analytics programs to ensure long-lasting success.
About BPI Partner APQC
APQC helps organizations work smarter, faster, and with greater confidence. It is the world’s foremost authority in benchmarking, best practices, process and performance improvement, and knowledge management. APQC’s unique structure as a member-based nonprofit makes it a differentiator in the marketplace. APQC partners with more than 500 member organizations worldwide in all industries. With more than 40 years of experience, APQC remains the world’s leader in transforming organizations. Visit us at www.apqc.org, and learn how you can make best practices your practices.